Mastering Pipeline Data Quality: A Critical Component of Effective Asset Management
In the complex world of pipeline operations, the quality of your data can make or break your asset management strategy. Poor data quality doesn't just lead to inefficiencies—it can compromise safety, compliance, and your bottom line. As regulatory scrutiny intensifies and operational margins tighten, can you afford to ignore the state of your pipeline data?
Watch this 30-minute ‘Ask SME a Question’ session as DNV’s Subject Matter Experts, Matt Julius and Alex Woll address your data quality issues and explore effective strategies. The interactive Q&A format goes beyond presentations and provides real-time solutions to your specific data challenges.
In this session, our SMEs answer questions such as:
The approach to this challenge differs between transmission and distribution assets. On the transmission side, TVC record verification efforts have helped validate data sources. A similar mandate is missing on the distribution side, where such a system.
would offer significant value but would also be a substantial undertaking. Without confidence in data at the record level, operators are somewhat limited in their ability to effectively use it.
Generally, more recent data tends to be more accurate and complete, but this represents only a small portion of the overall records. To mitigate this uncertainty, incorporating data validation into the analysis is essential to catch obvious data errors. For unvalidated data, operators can implement validation checks based on reasonable conditions for assets, use "most likely" and "worst case" scenarios in risk assessments and remember that having uncertain data is a risk that should be accounted for in risk models. |
Yes, it's common to use PODS (Pipeline Open Data Standard) within a GIS Esri system, especially for transmission pipeline operators. PODS provides a standardized structure for managing pipeline data, and when combined with Esri’s GIS platform, it offers a strong solution for organizing both spatial (location-based) and non-spatial pipeline information.
DNV’s Synergi Pipeline solution doesn’t replace PODS. Instead, it integrates with the PODS data model to offer more advanced capabilities, especially in analyzing pipeline integrity. By working with data maintained in a PODS database, Synergi Pipeline facilitates efficient data collection, aggregation, and analysis of pipeline integrity activities, ensuring that data is kept up-to-date and consistent.
For companies that manage both transmission and distribution pipelines (vertically integrated companies), DNV’s system can support more versatile data models like UPDM (Utility and Pipeline Data Model), which handles both types of assets in one database. This flexibility allows for streamlined management of various pipeline assets in a single system. |
The approach to this challenge differs between transmission and distribution assets. On the transmission side, TVC record verification efforts have helped validate data sources. A similar mandate is missing on the distribution side, where such a system would offer significant value but would also be a substantial undertaking. Without confidence in data at the record level, operators are somewhat limited in their ability to effectively use it.
Generally, more recent data tends to be more accurate and complete, but this represents only a small portion of the overall records. To mitigate this uncertainty, incorporating data validation into the analysis is essential to catch obvious data errors. For unvalidated data, operators can implement validation checks based on reasonable conditions for assets, use "most likely" and "worst case" scenarios in risk assessments and remember that having uncertain data is a risk that should be accounted for in risk models. |
It's difficult to draw a clear boundary when it comes to data quality confidence. Validated records are straightforward and can be used with confidence. For unvalidated records, the uncertainty can be addressed in risk assessments by running the model using "Most Likely" vs. "Worst Case" assumptions. If no validation effort has been conducted on the data, you're essentially left relying on it with limited validation. In cases where a record is clearly incorrect—like a plastic pipe showing a 1910 installation date— you can apply default values using the same "Most Likely" vs. "Worst Case" approach. The key is to avoid excluding assets from analysis due to lack of data; instead, handle the uncertainty within your risk logic. Remember that having bad data is a risk and should be accounted for in risk models. |
Data collected electronically and as close to the asset as possible tends to be of the highest quality. With proper controls in place, many data quality concerns can be addressed. This approach ensures timely integration into source systems, the use of input controls, and accurate spatial data collection, which ties the information to specific assets or coordinates. For bulk data acquisition, such as during pipeline acquisition projects, an initial audit is crucial to catalogue any data quality issues before incorporation. We apply a similar audit process before every project to establish a baseline understanding of the client's data quality. For new construction, it's advisable to use modern technology and robust data collection strategies. When dealing with acquisitions, running acquired data through a data audit process is essential. This can involve using commercial tools that automate data checks when available, as well as implementing internal checklists to verify data validity, geometry, measures, and other critical attributes. |
Managing data consistency over time is a crucial challenge, especially as operator needs evolve. An illustrative example of this is the change in client lists over time, particularly with the introduction of call confidence requirements with the implementation of API 1163. Schemas should be designed to change over time to adapt to real-world conditions and new requirements. When specific changes occur, such as the inclusion of new data points like call confidence for liquid transmission operators, operators need to consider whether to implement a consistent migration from old to new data formats or to sunset old records and transition entirely to the new format. The key is to ensure that your GIS remains a living representation of your operating conditions, adjusting schemas to accommodate new business requirements while maintaining historical context where necessary. |
As a rule, data sources should be electronic in nature and be consumable in a database-like manner. Formats that should be avoided or minimized include paper records, PDFs, images, and Excel spreadsheets (especially those formatted for human readability rather than data processing). These formats are often problematic because they require manual intervention to extract and process the data, which can be time-consuming and error prone. Instead, prefer formats that resemble database records, such as properly structured CSV files, database exports, or native GIS data formats. These are much easier to consume programmatically and integrate into automated data processing workflows. |
The importance of pipeline data quality cannot be overstated. It is as crucial as the decisions for which the data is being used to support. High-quality data forms the foundation for accurate risk assessments, effective integrity management, and informed decision-making across all aspects of pipeline operations. Poor data quality can lead to incorrect analyses, misguided resource allocation, and potentially compromised safety. As the industry moves towards more data-driven and proactive management approaches, the reliability and accuracy of the underlying data become even more critical. Investing in data quality is essentially investing in the overall safety, efficiency, and reliability of pipeline operations. |
To quantify "Bad Data" and assess its impact on data usability, the first step is to define what constitutes "Bad Data" and then identify it. One definition could be data that is clearly incorrect, as evidenced by conflicts within the data itself. For instance, a pipe with an installation year of 1910 but made of plastic is an obvious conflict. Establishing a process to detect such inconsistencies is crucial for resolving them.
If the definition of "Bad Data" expands to include low-quality records that lack proper validation, a process must also be put in place to flag those records. Once these problematic records are identified, the system using the data should be able to recognize and quantify the level of confidence in the data. This involves measuring data uncertainty, so users are aware of it when making decisions, and it helps prioritize efforts to improve data quality.
Ultimately, the goal is to create a structured process to detect and quantify "Bad Data," enabling the organization to understand the level of confidence in its datasets and to drive targeted improvements. |
Resources:
Meet our Pipeline Data Quality Experts
Matt Julias, Integrity Consultant, DNV
Matt has over 25 years of experience in Geographic Information Systems (GIS) and 12 years implementing Integrity and MRP solutions. Leveraging his expertise in environmental science, geography, and data management, Matt will be sharing his insights on how to improve pipeline data quality and address the challenges you may be facing.
Alex Woll, Head of Section - Risk Management, DNV
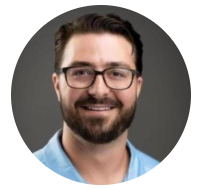
Watch this webinar to transform your Pipeline Data into a strategic asset.