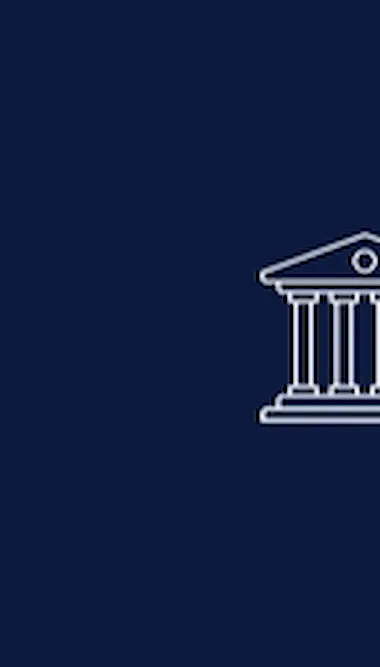
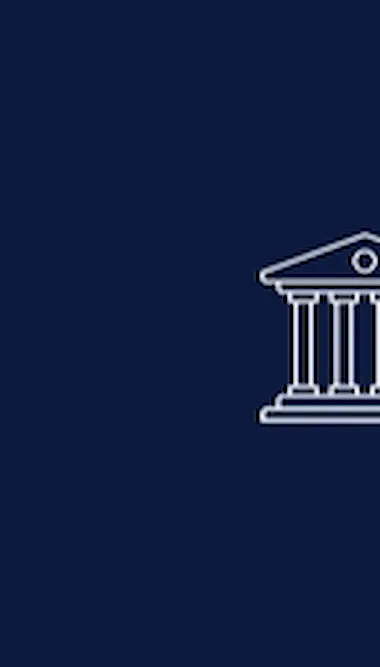
Navigating AI regulations: Key to fast adoption, competitive advantage and driving the energy transition
Artificial intelligence (AI) is driving transformative changes, enhancing efficiency, reliability and sustainability in the energy sector. However, the integration of AI into this critical industry makes stringent regulatory compliance necessary to ensure safety, ethical use and public trust.
The introduction of new regulations specifically targeting AI and data management such as the EU AI act marks a significant step towards responsible AI development and deployment in the energy industry. By addressing data protection, ethical use, transparency, sustainability, and international coordination, these regulations help ensure that AI technologies are used in ways that benefit society and the environment.
Many organizations are struggling to navigate the complexities of the changing regulatory environment while also taking advantage of the potential opportunities of AI. Understanding regulatory requirements and identifying potential compliance gaps are essential steps. Organizations must also establish appropriate governance and compliance frameworks to ensure responsible AI adoption.
How DNV can help to prepare for regulatory and standards compliance
Employing recognized industry standards, frameworks and DNV methodologies, we provide tailored advice on how to prepare for regulatory compliance and meet recognized industry standards so you and your stakeholders can adopt AI with confidence, faster.
Identify the AI standards and regional/national regulations that apply to your organization and industry and get guidance on how to prepare for compliance. |
It can be challenging to keep track of a changing, complex regulatory environment. Our experts work closely with authorities and regulatory bodies to be on top of regulatory development and can provide you with expert guidance on the latest developments. |
Our experts can conduct a comprehensive technical evaluation of your AI solutions, assessing their compliance with the EU AI Act and identifying the steps needed to meet its requirements. |
Many regulatory frameworks, such as the EU AI Act, mandate that AI systems must be explainable. Regulations often set specific safety and performance standards that AI systems must meet. Many AI systems operate as “black boxes,” where the internal workings are not visible or understandable to users. AI explainability and reliability testing are fundamental to the responsible development and deployment of AI technologies. Explainability builds trust, ensures accountability, facilitates regulatory compliance and enhances user understanding. Reliability testing ensures safety, mitigates risks, supports continuous improvement and ensures compliance with industry standards. |
In response to the rise of Artificial Intelligence (AI), the ISO and IEC have created the ISO/IEC 42001 standard. Certification of your management system demonstrates your commitment to consistency, continual improvement and customer satisfaction. Also, a certified system based on the new ISO/IEC 42001 standard helps any company ensure reliable and responsible use to safeguard all people involved and build trust in its application. Learn more about ISO/IEC 42001 Certification with DNV here. |
This does not constitute legal advice. DNV recommends that you seek specific legal advice for your circumstances.
Why partner with DNV?
Meet some of our AI experts:
Featured articles and customer stories:
Recommended practices:
On-demand webinars:
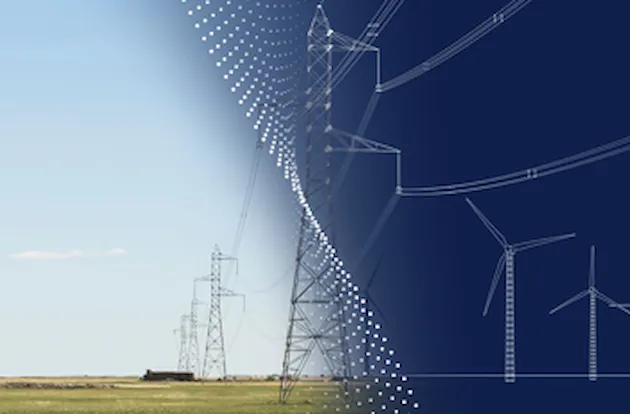
How can the energy sector get real value from industrial AI?
Watch the webinar discussion
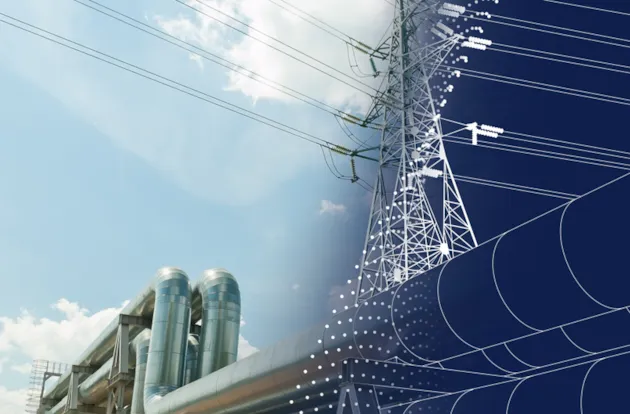
How to ensure trust and compliance in an AI-driven energy supply chain
Watch the webinar discussion
Read our frequently asked questions about Artificial Intelligence (AI):
Artificial intelligence (AI) is a common designation of technologies where a machine performs tasks that are considered to require intelligence. This typically relates to speech recognition, computer vision, problem solving, logical inference, optimalizations, recommendations, etc. |
AI is often divided into two main domains: Rule-based AI and machine learning. Rule-based AI is where we take human insight and knowledge and codify it into rules, such that the machine can perform tasks based on these rules. This kind of AI is very structured and explainable, but less flexible, as it can only be used for tasks for which specific rules have been developed. Machine learning (ML), on the other hand, is AI which is created from data. The applications infer their own rules and correlations from the data. This makes for flexible models, but with larger ML models, it can be difficult to explain decisions. In many practical applications, a combination of rule-based and machine learning is used. |
The EU AI Act is a new regulation of AI use in the European Union. The Act’s purpose is: ‘To improve the functioning of the internal market and promote the uptake of human-centric and trustworthy artificial intelligence (AI), while ensuring a high level of protection of health, safety, fundamental rights enshrined in the Charter of Fundamental Rights, including democracy, the rule of law and environmental protection, against the harmful effects of artificial intelligence systems (AI systems) in the Union, and to support innovation.’ The Act sets a common risk-based framework for using and supplying AI systems in the EU. It is binding on all EU member states and requires no additional approval at national level. However, variations on how national regulatory bodies are set up, and guidelines on how to align with other member states’ regulations, and so on, will be established. Learn more on EU’s official pages here. |
The EU AI Act regulates all AI in Europe. To understand what is required, one must first assess the risk category of the AI. Learn more on EU’s official pages here. |
The EU Act passed the EU Parliament in March 2024, and will entry into force June 2024. There is in general a 2-year period until compliance must be in place. But there are also earlier statutory milestones along the way. For example, after 6 months of the Act coming into force, a ban on prohibited AI practices must be in place. Rules on General Purpose AI (GPAI) are required after 12 months. Obligations for high-risk systems must be in force within 24 months. Learn more on EU’s official pages here. |
High-risk AI means that the supplier and deployer (user) must meet stringent regulatory requirements for use. Providers of a high-risk AI system will have to put it through a regulatory conformity assessment before offering it in the EU or otherwise putting it into service. They will also have to implement quality and risk management systems for such an AI system. Learn more on EU’s official pages here. |
Generative AI is a type of machine learning that can create new data (numbers, text, video, etc) from an underlying data distribution. Generative AI is therefore probabilistic in nature. |
Conformance testing (or compliance testing) means to test a system to assess if it meets given standards or specific requirements. |
Fairness of an AI system is often defined to mean that the AI system does not contain bias or discrimination. This means that the AI system is created from data that are representative for the kind of distribution and algorithmic behaviour we would want the AI system to have. |
Algorithm verification means to assess if an algorithm meets specific requirements. When AI is deployed into larger systems, we need to assess how the system and its components work. Algorithm testing is one way of verifying that the AI algorithm works as intended. |
AI assurance means to (i) establish what requirements the AI needs to meet and (ii) verify compliance to these requirements. |
The AI lifecycle covers all the phases of AI, from problem definition to data acquisition, to model development, deployment and update. The lifecycle is often iterated several times. |
Model validation means to ensure that the model is solving the right problem, by comparing model outputs to independent real-world observations. Without a validated model you cannot trust that the models are solving the right problem. |
Black-box testing means testing of a model without access to or insight into its internal structure or working. Inputs are provided to the black-box and outputs are received. |