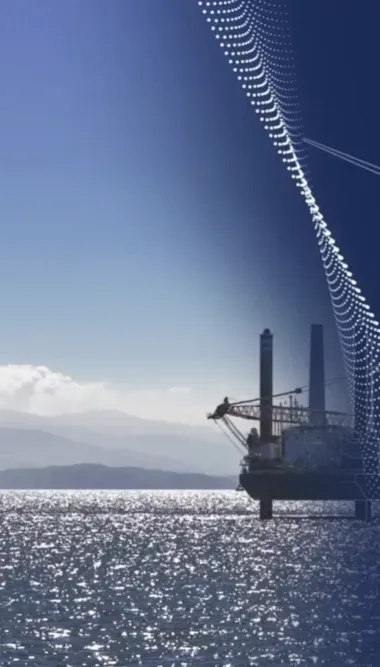
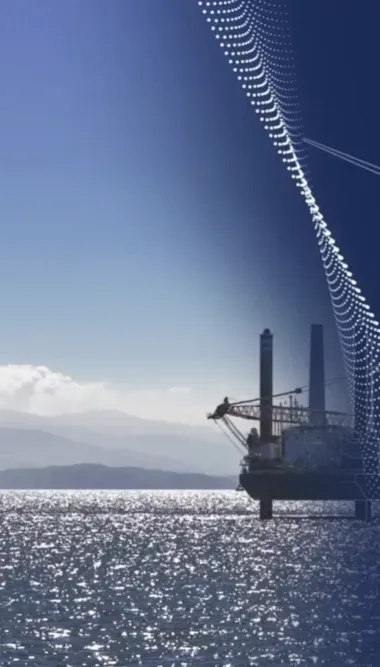
Challenges and opportunities of trustworthy and responsible AI in the energy industry
The energy industry is making substantial investments in artificial intelligence (AI). Their goal? To enhance operational efficiency, optimize maintenance practices, and maximize asset utilization—all while meeting the growing demand for safe, affordable, and sustainable energy supply. However, despite these efforts, many organizations grapple with demonstrating the trustworthiness and value of AI to stakeholders.
Recent research on digitalization in the energy industry reveals that only 14% of energy executives report being in an advanced stage of AI development or having live AI projects, while 70% report being in earlier stages including planning or piloting AI projects. Why this hesitancy? One contributing factor is the absence of clear guidelines and standards for implementing AI in a secure and reliable manner. The lack of a roadmap often leaves decision-makers uncertain about how to proceed.
At DNV, we recognize the trust gap between expectations and actual value realization from AI. Our portfolio of Digital Trust services and recommended practices aims to bridge this gap, ensuring that organizations can confidently leverage AI for sustainable energy solutions.
Source: DNV Energy Industry Insights 2024: Leading a data-driven transition
Our services for responsible and trustworthy industrial AI
Taking industrial AI from hype to real value
AI regulations and standards compliance
Prepare for regulatory compliance and ensure your business meets relevant industry standards with expert guidance, comprehensive assessments, and tailored compliance strategies.
Industrial AI governance and strategy advisory
Equip your business with tailored AI strategies, robust governance, and seamless implementation to confidently navigate your AI journey.
Meet our AI experts
-
Dr Frank Børre Pedersen
Vice President and Programme Director in Group Research and Development, leveraging extensive technical and managerial experience across oil & gas, maritime, and renewable energy domains, driven by his passion for integrating technology understanding with practical applications to meet customer needs.
-
Dr Carla Janaina Ferreira
Dr Ferreira has extensive experience in assessing the uncertainty of machine learning models and their integration with domain-specific knowledge, ultimately influencing critical decision-making processes. She is passionate about building confidence in model-based predictions though the combination of physics- and data-driven models.
-
Dr Abdillah Suyuthi
Head of Machine Learning services leveraging extensive industry experience in executing simulation model projects, creating trustworthy machine learning solutions and developing efficient methods and tools, with a passion for data quality, integration of large language models and ontologies to propel progress and foster sustainability.
-
Dr Stephanie Kemna
Principal Research Scientist in the Digital Assurance Program in Group Research and Development leveraging extensive experience developing adaptive behaviours, autonomy and mission planning for aquatic robots, including autonomous underwater vehicles (AUVs) and Autonomous Surface Vehicles (ASVs). She is passionate about working with AI and robotics, particularly on the development of autonomous systems.
-
Dr Christian Agrell
Dr Agrell leverages extensive experience within developing trustworthy AI, particularly for high-risk and safety-critical systems in an industrial context. He is driven by a passion for the intersection of machine learning, uncertainty quantification, physics based and data-driven simulation, assurance of complex systems and risk.
-
Dr Sara El Mekkaoui
Dr El Mekkaoui has a strong background in machine learning especially within the maritime industry and has extensive experience in shipping and logistics. She is passionate about leveraging advanced technologies to solve complex, real-world problems and enhance safety and efficiency.
-
Martine Berglund Hannevik
Martine has a strong background in organizational change and as a leader of innovation projects, especially within the energy and maritime industry. She is passionate about innovation, digital transformation, leadership and change.
Recommended practices
Featured articles
Recorded webinars
Webinar: How can the energy sector get real value from industrial AI?
Watch the webinar discussion
How to ensure trust and compliance in an AI-driven energy supply chain
Watch the webinar discussion
Case studies
Explore more about Artificial Intelligence
AI Insights
Get insights, resources, and advice on trustworthiness and compliance for AI designers and developers and organizations deploying or using AI systems.
AI Research and Development
Learn more about how we work on understanding how is AI regulated and how these technologies can be assured in order to be trustworthy.
Read our frequently asked questions about Artificial Intelligence (AI):
Artificial intelligence (AI) is a common designation of technologies where a machine performs tasks that are considered to require intelligence. This typically relates to speech recognition, computer vision, problem solving, logical inference, optimalizations, recommendations, etc. |
AI is often divided into two main domains: Rule-based AI and machine learning. Rule-based AI is where we take human insight and knowledge and codify it into rules, such that the machine can perform tasks based on these rules. This kind of AI is very structured and explainable, but less flexible, as it can only be used for tasks for which specific rules have been developed. Machine learning (ML), on the other hand, is AI which is created from data. The applications infer their own rules and correlations from the data. This makes for flexible models, but with larger ML models, it can be difficult to explain decisions. In many practical applications, a combination of rule-based and machine learning is used. |
The EU AI Act is a new regulation of AI use in the European Union. The Act’s purpose is: ‘To improve the functioning of the internal market and promote the uptake of human-centric and trustworthy artificial intelligence (AI), while ensuring a high level of protection of health, safety, fundamental rights enshrined in the Charter of Fundamental Rights, including democracy, the rule of law and environmental protection, against the harmful effects of artificial intelligence systems (AI systems) in the Union, and to support innovation.’ The Act sets a common risk-based framework for using and supplying AI systems in the EU. It is binding on all EU member states and requires no additional approval at national level. However, variations on how national regulatory bodies are set up, and guidelines on how to align with other member states’ regulations, and so on, will be established. Learn more on EU’s official pages here. |
The EU AI Act regulates all AI in Europe. To understand what is required, one must first assess the risk category of the AI. Learn more on EU’s official pages here. |
The EU Act passed the EU Parliament in March 2024, and will entry into force June 2024. There is in general a 2-year period until compliance must be in place. But there are also earlier statutory milestones along the way. For example, after 6 months of the Act coming into force, a ban on prohibited AI practices must be in place. Rules on General Purpose AI (GPAI) are required after 12 months. Obligations for high-risk systems must be in force within 24 months. Learn more on EU’s official pages here. |
High-risk AI means that the supplier and deployer (user) must meet stringent regulatory requirements for use. Providers of a high-risk AI system will have to put it through a regulatory conformity assessment before offering it in the EU or otherwise putting it into service. They will also have to implement quality and risk management systems for such an AI system. Learn more on EU’s official pages here. |
Generative AI is a type of machine learning that can create new data (numbers, text, video, etc) from an underlying data distribution. Generative AI is therefore probabilistic in nature. |
Conformance testing (or compliance testing) means to test a system to assess if it meets given standards or specific requirements. |
Fairness of an AI system is often defined to mean that the AI system does not contain bias or discrimination. This means that the AI system is created from data that are representative for the kind of distribution and algorithmic behaviour we would want the AI system to have. |
Algorithm verification means to assess if an algorithm meets specific requirements. When AI is deployed into larger systems, we need to assess how the system and its components work. Algorithm testing is one way of verifying that the AI algorithm works as intended. |
AI assurance means to (i) establish what requirements the AI needs to meet and (ii) verify compliance to these requirements. |
The AI lifecycle covers all the phases of AI, from problem definition to data acquisition, to model development, deployment and update. The lifecycle is often iterated several times. |
Model validation means to ensure that the model is solving the right problem, by comparing model outputs to independent real-world observations. Without a validated model you cannot trust that the models are solving the right problem. |
Black-box testing means testing of a model without access to or insight into its internal structure or working. Inputs are provided to the black-box and outputs are received. |