Building trust in data for AI
The expression ‘garbage-in, garbage out’ describing the outcome of relying on ‘bad’ data has been used since the 1950s but has never been more appropriate than today, given our vastly greater computing power and reliance on it.
The volume of data in the energy system is huge and growing rapidly – for example, with digital and smart metering in power grid systems. Reliance on data is also being driven now by people and organizations designing, training, testing, and applying artificial intelligence (AI) and its subsets machine learning (ML) and deep learning.
The use of AI is progressing in the context of increasing industrial digitalization to enable and manage decarbonization and decentralization (e.g. distributed power generation) to further the energy transition that we need to mitigate global warming.
Industries need to be able to trust that their digital hardware and software technologies and systems will not malfunction, potentially creating risks to health, safety, assets, profitability, the environment, and energy security. The need for trust is amplified, not least through regulatory requirements, when it comes to critical infrastructure such as power grids and pipelines.
Consequently, it is essential to be able to trust the data which ‘fuels’ IT, operational technology (OT), and any associated AI. Ensuring ‘data quality’ is fundamental to creating such trust.
Senior energy industry professionals recognize this. Three-quarters (74%) of more than 1,300 surveyed for DNV’s Energy Industry Insights 2024 report said their organizations would be prioritizing improving data quality and availability in the year ahead. They ranked digital quality management as the third biggest barrier to digitalization (Figure 1).
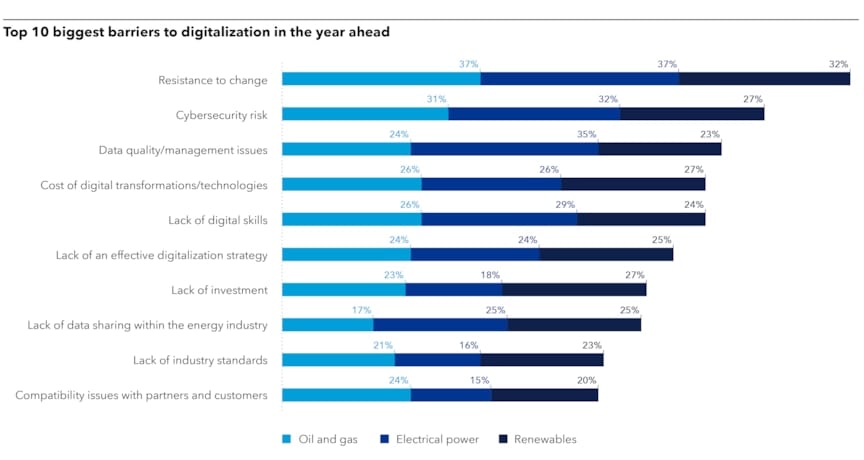
What is data quality?
Beyond establishing the properties of data, each intended use of it should come with a checklist of quality criteria and thresholds for the data to comply with. The criteria and thresholds are not fixed forever and may have to change if the use does; data and models, including AI/ML, have life cycles. The key point is that only when data quality can be measured can organizations be sure that it is fit for purpose. What can be measured can be improved, hence the need for data quality diagnostics, monitoring, and improvement.
Hence, assuring data quality involves the measurement of the degree to which data meets the implicit or explicit expectations and requirements of users or systems utilizing the data. Information and data quality is defined and measured according to syntactic, semantic, and pragmatic quality (Figure 2).
Syntactic and semantic quality is measured through a verification process, whereas pragmatic quality is measured through a validation process. Verification describes the confirmation, through the provision of objective evidence, that specified requirements have been fulfilled. Validation describes the confirmation, through the provision of objective evidence, that the requirements for a specific intended use or application have been fulfilled.
Key data quality assurance considerations for AI/ML include accuracy, bias, completeness, consistency, data volume, integrity, relevance, security and privacy, timelines, and uniqueness.
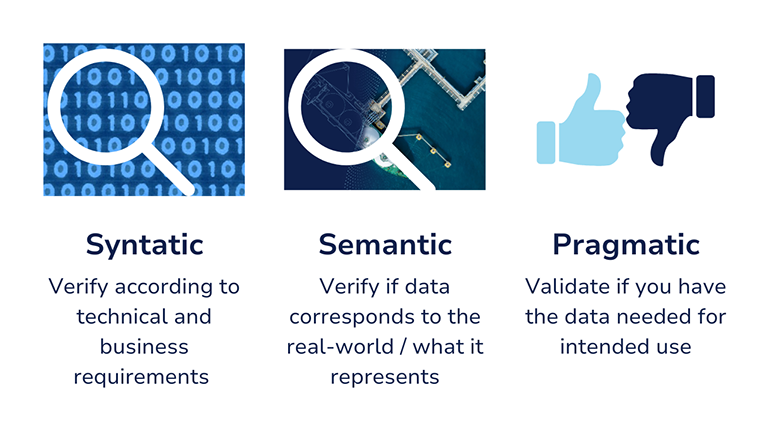
Connectivity brings new data quality challenges
Connectivity is shifting the needle on data quality. Many legacy IT and OT systems have operated in isolation but are now connecting to others in and beyond the organization. In some cases, their data output is input for secondary purposes. For example, monitoring and control systems aim to observe and record data about a physical system using sensors. This data is primarily utilized to control the system’s behaviour. When correctly implemented, a secondary purpose of such data serves as input for predictive maintenance systems.
Organizations harvesting external data from others, or from open data, must ensure that their own data is fit for secondary purposes and for sharing with other organizations.
Building trust in data is paramount for the data sharing that can unlock AI’s potential benefits for energy industries, namely cost savings and quicker and better decision-making to optimize operations, levels of service, and the management of more complex networks and dealing in more sophisticated markets.
Data quality for AI
Data quality is important for being able to trust artificial intelligence technologies, whether they are discriminative or generative AI.
Discriminative AI models focus on identifying patterns and making decisions based on them. They are used for predictions and inferences based on existing data, but do not generate new data. Hence, the quality of conclusions and decision-making based on discriminative AI hinges on the quality of the data input.
Generative AI models generate new data that aims to learn the underlying distribution of the data to produce new samples. One main barrier to generative AI’s uptake is its tendency to ‘hallucinate’. If there is no or flawed data as input, generative AI may just make things up like someone imagining that any answer to a question is better than none.
The risks in using these technologies in critical industrial applications are magnified by the ‘black box’ nature of much AI. If users do not know or cannot see how it works, they may just trust its output anyhow, with potentially negative safety, economic, societal and political consequences.
Data ‘quality’ is hence a central issue in being able to trust AI so that its benefits can come with levels of risk that comply with regulation and in some cases go beyond to meet business objectives. As AI/ML becomes increasingly sophisticated and connected, it is important to understand each system element’s dependence (e.g. low to very high criticality) on data quality.
Regulatory compliance of data quality
Compliance is not an abstract issue; regulators are now targeting data quality for AI. For example, data quality and management requirements are included in the EU AI Act (2024) as applied to providers of high-risk AI that is or will be used in the EU.
Broadly speaking, examples of ‘high risk’ included in this Act include AI usage that can have severe negative consequences if it fails; AI to be used in critical infrastructure; AI use for key/critical cyber-security functions; and vendors of AI services, AI products, or systems/products with AI inside. Those wishing to understand the detailed ramifications for their own organizations should consult their own legal departments or advisers.
Organizations using or planning to deploy AI need a formal framework and process to answer key questions in preparing for regulatory compliance in whatever jurisdiction it applies. These questions include, among others, what data quality and management criteria the organization’s data must meet to satisfy legislative requirements.
However, business needs to extend data quality considerations beyond simply complying with regulations, and this poses a different set of questions.
What value will using AI deliver to customers? What data quality is needed to succeed in using AI to maintain or boost competitiveness? What should the sales messaging around AI be? As AI/ML becomes increasingly regulated, what balance might there be between risks and benefits of describing a product to be AI-related if legal advice suggests that regulations do not require such a declaration?
Assuring data quality management
Data quality for AI is most powerful when conducted within and alongside complementary organizational strategies for AI, data, and digitalization. Any digitalization journey should include a focus on improving data quality and ensuring robust data governance processes to ensure trust in data for critical decision-making.
The same applies to implementing an organization’s AI strategy, which is why DNV published Recommended Practice DNV-RP-0497 for assurance of data quality management, for its own and its customers’ use.
DNV-RP-0497 provides a framework to audit and validate data against international benchmarks. It is one among a set of DNV recommended practices for digital applications (Figure 3).
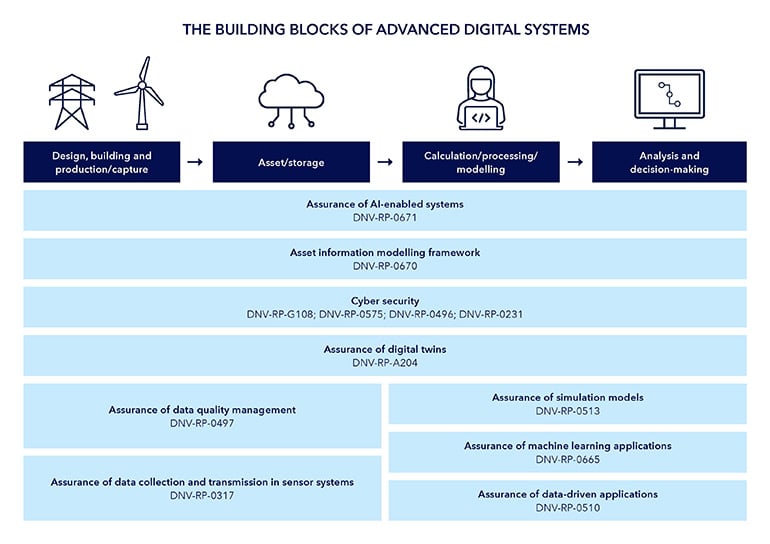
Is your organization ready for AI?
Organizations with a high level of readiness for AI are believed to have a higher probability of success with their AI investments. So, DNV is also launching an AI readiness survey process to support customers from early in their AI journey.
The process assesses how an organization – including all its people, resources, and technology – is set up to harness its AI opportunities. Data quality and management are included in the criteria.
The questions and topics in the survey are inspired and based on best practices, current legislation, and international standards. The readiness questions relate to characteristics of the AI system deployer using an AI system under its authority; or, of a provider that develops or puts the AI system into service; and, of the AI system and its resources.
The survey can be combined with other DNV self-assessment surveys such as data management maturity, cyber-security maturity, digital twin maturity, ISO 42001 AI management system self-assessment, and others.
Learn more about DNV's data quality services
9/30/2024 12:47:00 PM